In the first article of our AI performance series, we’ve tackled the key indicators and why they matter. In this second article, we will walk you through the Real-World Strategies for Field Services to continuously measure and refine AI performance to keep it aligned with your business goals and deliver maximum impact in field services.
Measuring AI Performance In Pre-Production
The pre-production phase lays the foundation for the entire AI system. At this stage, data scientists are used to assess the quality of deep learning models, making adjustments when necessary to meet performance expectations.
The next step is to test the waters to get a feel for its efficacy. This is done in controlled conditions which allow users to identify potential gaps and attend to them swiftly.
Errors are identified and amended prior to its launch, ensuring that the technology is firing on all cylinders when released into the field.
What does this lead to?
Fewer errors and less time wasted in the field, resulting in significant cost savings.
Ground-Truth Datasets
Ground-truth datasets serve as a benchmark for evaluating machine learning model performance. They’re essentially the barometer of AI performance and function by accurately labeling data that represents the real-world conditions that the artificial intelligence will encounter.
Example: A field service company using AI to detect power line defects
Data scientists collect and label images of power lines with and without defects. These ground-truth datasets provide AI models with context, helping them visualize what’s correct and what’s wrong.
The AI is then tested against data, giving it an opportunity for its predictions to be compared with real-world scenarios. Data scientists can then measure the model’s accuracy, identify errors, and make adjustments accordingly.
Without ground-truth data sets, the AI will be flying blind in its analysis, unsure of what’s correct and what’s false. Inevitably, this leads to a slew of errors that jeopardize the safety and accuracy of on-field operations.
Collaboration with Customers
It’s important to underline that ground-truth datasets should not be built in isolation without customer feedback. Why is this important?
Each on-field company has its own set of needs and requires the AI to identify specific characteristics to reach accurate, relevant conclusions. Including customer feedback provides AI models with a complete picture of what serves as the benchmark.
At Deepomatic, our client collaborations are crucial for optimizing AI for quality control because it ensures that datasets reflect the specific challenges and quality standards of the client’s operations. This allows the data scientists to tweak the AI until it is in concordance with the customer’s expectations.
Here’s a matrix we’ve created to help you visualize the distribution of responsibilities between the provider and the customer.
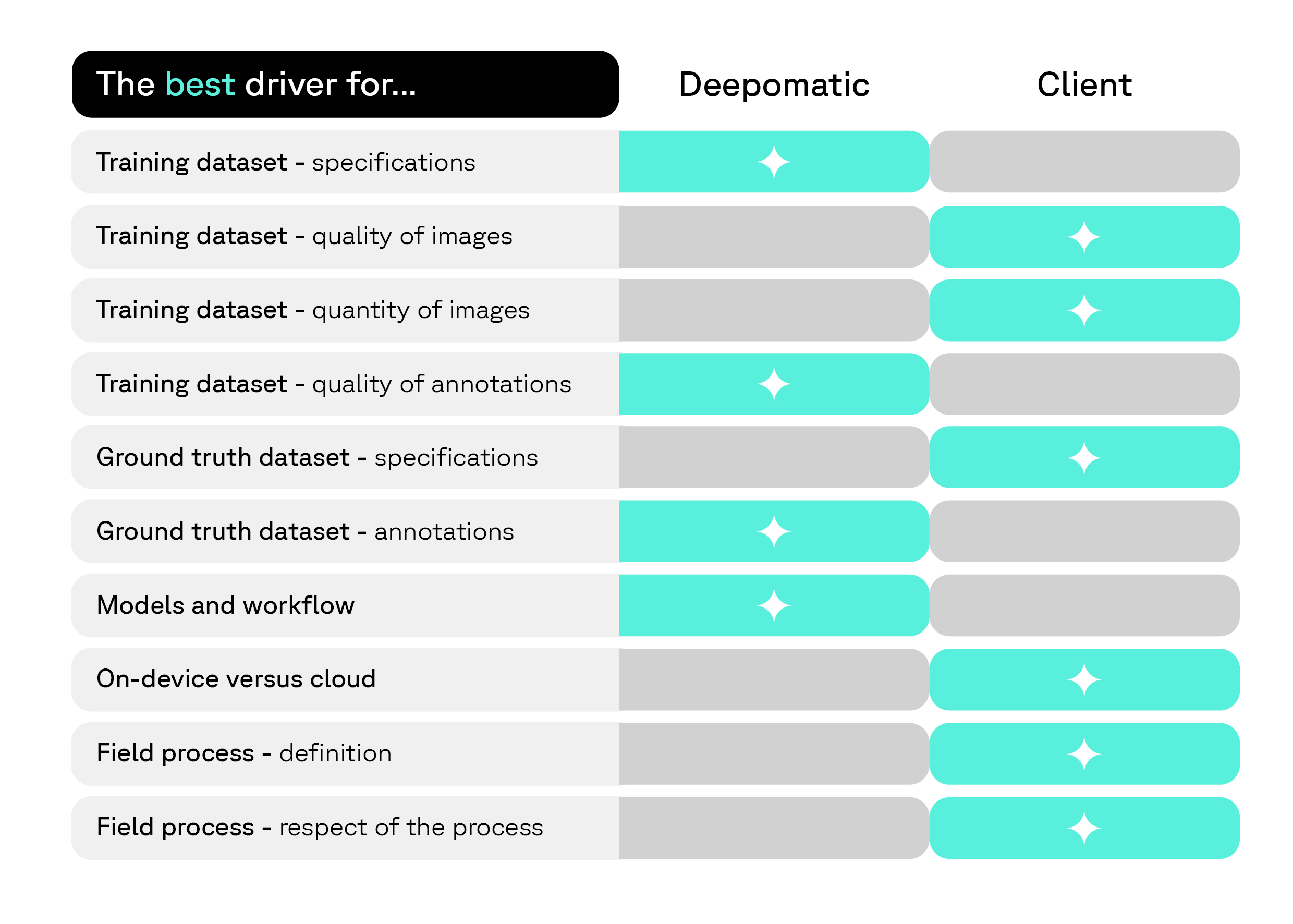
Through this mutual understanding, data scientists can effectively lay the groundwork for a smoother deployment and a system that delivers maximal value.
Acceptance Tests
The foundation has been laid. Now it's time to assess the AI’s performance through a series of acceptance tests. We’re transitioning from the theoretical to the practical. Acceptance tests evaluate the AI’s readiness by inserting it into real-life scenarios under specific conditions.
Achieving this involves placing the AI systems silently at first through a series of methodical steps:
- Initial Silent AI Analysis: Field technicians take photos and feed them to the AI system without providing any feedback. The AI’s intuition is tested, and it delivers a result that is internally assessed by the team, allowing us to evaluate its performance in real-world conditions without influencing the technician’s actions.
- Feedback: Feedback is introduced gradually, starting with alerts that don't interrupt workflow (non-blocking warnings), allowing technicians time to adapt to the AI system during a controlled transition.
- Gradual Rollout of Checks: Different types of checks are progressively activated, starting with basic image quality and fraud detection. More advanced context validation is followed for a comprehensive evaluation.
With this process, AI models can naturally evolve efficiently and reach a level of efficacy.

Production: Monitoring AI in Real-Life Conditions
In the production phase, AI systems are continuously monitored to ensure that every facet of their analysis is on-point. This real-time quality control and performance management process is carried out in real life conditions by a team of annotation specialists.
The review and the computed metrics are made available through dashboards so that everyone can see the evolution of the performance.
AI Performance Metrics To Observe
Assessing AI performance requires you to primarily focus on what are the expected outcomes based on specific use-case. Here are some examples:
- Fraud Detection & Image Quality: Ensures the AI accurately detects issues while minimizing false positives, providing feedback only when highly confident. (Metric that matters most is high precision)
- Safety Defect Detection: Prioritizes detecting all potential safety threats, even if some false positives occur, to prevent high-risk incidents. (Metric that matters most is high recall)
- Patch Panel Inventory: Focuses on gradually improving accuracy in identifying patch panel components, reducing service disruptions, and enhancing customer experience.
- Human-in-the-Loop Quality Control: Involves human oversight for complex cases, allowing technicians to override AI as needed to maintain high quality standards.
With these metrics at the forefront, you can gauge the efficacy of your AI systems to ensure that they’re delivering reliable results that are aligned with your operational goals.
Overcoming AI Underperformance
You’ve deployed your models and now performance levels are subpar. What do you do now? When this happens, you can make a number of strategic adjustments to refine their accuracy and reliability:
- Confidence Score Strategy: Adjusting AI confidence scores can balance recall and precision, ensuring predictions are only provided when confidence is high, while lower-confidence cases are escalated for human review.
- Silent Mode Deployment: When AI performance is low, deploying in silent mode (where feedback isn’t given to technicians) allows the system to gather data and refine its accuracy without impacting workflows.
- Task Simplification: Breaking tasks into smaller components or simplifying processes can improve AI accuracy. For example, using multiple photos from different angles can help modern AI systems capture more relevant data, boosting overall performance.
Using Deepomatic, you can implement these strategies seamlessly, allowing your AI models to continuously improve and adapt to real-world conditions. Deepomatic’s tools give you the ability to make these strategic adjustments with ease, providing the agility to quickly address and recover from any areas of underperformance.
Continuous Monitoring Is The Key to Maximizing AI Performance
AI has a wealth of potential to elevate the efficiency and accuracy of on-field operations. But to maximize AI performance levels, it must undergo continuous monitoring, adjustment, and collaboration.
The pre-production phase lays the groundwork for smooth AI deployment, ensuring that the entire system is tested and aligned with specific operational goals before it’s used in real-world scenarios.
This ensures that every component of the system surpasses the minimum requirements for deployment. From here, ongoing monitoring, strategic adjustments, and the use of targeted AI metrics mold the system into a reliable, high-performing tool that adapts to real-world conditions.
Not only will you enhance AI capabilities but you’ll maximize employee performance, helping technicians to execute on-field tasks with greater precision and ease.
Want to know how Deepomatic Can Help You Measure and Improve AI Performance?