Over the past year, there has been a noticeable surge in advancements within the realm of Artificial Intelligence. This surge, more prominent than ever before, began with the introduction of image generation systems like Stable Diffusion and Midjourney, followed by the remarkable ascent of Generative AI, exemplified by OpenAI's ChatGPT. These advances led to intensified competition and the development of improved models, which were integrated into numerous major products by the end of May 2023, supported by an active open-source community.
In this article, we aim to delve into the AI branch that Deepomatic capitalizes on for its platform: computer vision. We will explore its evolution since its inception, highlight emerging trends, and shed light on the potential of multimodal models to revolutionize quality control in field operations.
Understanding computer vision
Computer vision is a domain of Artificial Intelligence that automatically processes and analyzes digital images and videos to understand their meaning and context. Computer vision features a wide spectrum of capabilities, such as classification, tagging, detection, segmentation, and Optical Character Recognition (OCR). These tasks are known as "supervised learning" algorithms: they are "trained" from examples of annotated images with the concepts they should be able to recognize to predict the interesting concepts in images that the algorithm has never encountered before.
The evolution of Computer Vision applications
The first applications leveraging computer vision can be traced back to the 1960s and 1970s:
- Industrial inspection for quality control and defect detection
- Medical imaging for X-ray and CT scan analysis
- Robotics and Automation enabling robots to perceive and interact with their environment.
- Traffic control and surveillance encompassing tasks such as traffic monitoring, license plate recognition, and surveillance of high-security facilities
- Face recognition
In the past decade, new use cases have emerged, allowing diverse industries to harness the power of computer vision:
- Self-driving cars
- Smart checkout systems
- Quality control in the realm of field services. This latter application aims to assess and evaluate the quality and accuracy of the work performed by technicians in the field. If you are curious to read more about it, download our FieldForce Empowerment whitepaper.
Single-modal models
Until recently, computer vision relied on single-modal models, which operate exclusively on data from a single source or modality, such as images. A traditional image classification model, like a Convolutional Neural Network (CNN), is an example of a single-modal model. It takes 2D image data as input and learns to recognize patterns and features within these images to classify them into predefined categories.
Single-modal models have limitations. Indeed, if we look at the way humans experience the world, it is through a combination of objects, sounds, textures, smells, and flavors. For AI to make progress in understanding the world around us, it needs to be able to interpret such multimodal signals together, just like we do.
Single modal models are trained to only one specific set of tasks. Therefore, they offer a limited level of configuration and adaptability, and they require systematically to collect data and train the models. This is why, today, multimodal models are emerging.
Transitioning to Multimodal Models
Multimodal machine learning models can handle data from multiple modalities: image, text, audio, video, temperature, depth, etc., to gain a deeper understanding of content. They allow the learning of more complex relationships and patterns across different types of inputs. The most popular combinations are:
- Image + Text
- Image + Audio
- Image + Text + Audio
- Text + Audio
An example of powerful multimodal model is ‘Flamingo’. Flamingo is a family of visual language models (VLMs) designed for few-shot learning. By providing a few examples to the learning model as a prompt, along with a query input, the model is able to generate a continuation to produce a predicted output for the task on the query.
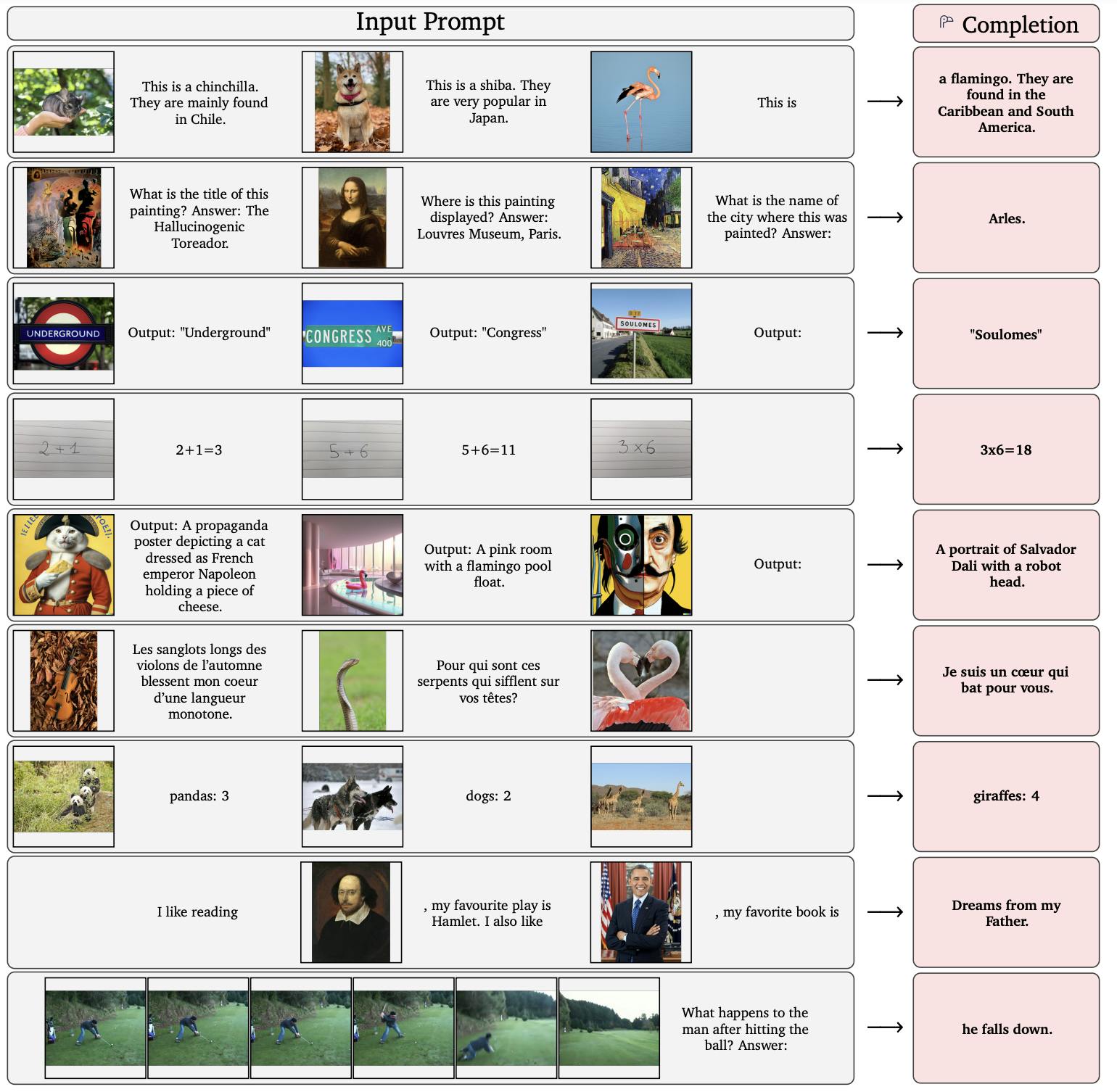
Examples of inputs and outputs obtained from 80B parameter Flamingo model
Applications in field services quality control
Field Operations and Infrastructure Lifecycle managers can retrieve operations more easily by interrogating the database of operations photos using textual questions like “Show me operations with missing stickers on the device, with unplugged cable” etc. This database serves as a robust search engine, providing access to powerful business intelligence derived from multiple sources of field data that can be crossed and interpreted.
When on-site, field workers retrieve information about similar equipment as the one they are working on in order to see how they can best do their tasks. This helps them improve their diagnosis of situations they find themselves in.
Benefits of Multimodal Models in Field Quality Control
How do multimodal models enhance field quality control efficiency?
Firstly, they ease the AI model configuration during the implementation by shortening the data collection phase. Indeed, companies no longer need large datasets to train the algorithms and can instead rely on few-shot learning.
Secondly, in terms of quality control analysis, multimodal models enable a more granular description of situations in the field. By crossing different types of data, they surpass a binary interpretation of photos taken by field workers, moving beyond a simple Yes or No approach to quality control. Therefore, multimodal models offer the advantage of removing the ambiguity that sometimes exists when leveraging only images. As a result, AI models deliver a more subtle and nuanced analysis of the context in which the job was carried out on the field. Moreover, live feedback provided to technicians can contain more information when a checkpoint is not validated to help the worker understand why it did not pass. For instance, if the installation of a heat pump at a customer’s house is unvalidated due to improper positioning, the solution would provide additional guidelines specifying that the pump should be located more than 40 cm away from any wall.
Additionally, multimodal models can assist in reducing false positives and false negatives, as information from one modality can compensate for any shortcomings in another.
Exploring New Frontiers in Field Multimodal Computer Vision
Multimodal models open up new possibilities to empower field companies with more AI capabilities. They can create complete textual diagnosis by leveraging the vocal feedback recorded by a field worker, as well as a video of the piece of equipment. They could also use a video of a fiber optic trench as input, and a 3D mapping of pieces of equipment that are laid inside as output, as well as any area of interest in it, and the automated quality control of fiber works in the form of text.
Another evolution to watch out for is the miniaturization of multimodal models. This consists in having them run on smartphones. However, it requires making them less energy-intensive to ensure they can be used on the field by technicians and workers.
Limitations and the path forward
While in the past, we used to train various models to serve different types of industry-specific or job-specific purposes, but thanks to multimodal models, this training happens only once and aims at a more general purpose. However, these models can only solve complex problems if we train them on a large volume of industry-specific or job-related datasets. This is where Deepomatic comes into play, drawing on our expertise in quality control for field operations. We have accumulated extremely large datasets on specific industry verticals, and we are currently training vertical foundation models that will be able to solve a wide array of problems without further training.
The rise of multimodal computer vision models marks a significant advancement in the realm of quality control automation for field services. By harnessing the power of diverse data sources, these models offer a more sophisticated understanding of field situations, ultimately enhancing the efficiency and accuracy of quality assessments. As this technology continues to evolve, we anticipate further breakthroughs in the field of AI-driven quality control. Deepomatic's expertise in this domain, coupled with extensive industry-specific datasets, positions it at the forefront of this transformative journey.