Computer vision can transform the way fiber optic network and utilities providers run their field operations. Today, most providers don’t have a reliable way to gather and validate information about their networks and infrastructure out in the field. They rely on manual reporting processes and forms that technicians have to fill out while on the job site. Mistakes that a technician makes in the field—either in the data entry process or in the actual build or installation—can only be addressed with a costly site revisit. With a computer vision solution like Deepomatic, companies can leverage photos and automation to check the work their technicians are doing in the moment and get real-time insight into asset use and network quality.
Only 8% of enterprises in the EU are using AI in their business and just 2.2% are leveraging image recognition technology. There’s ample opportunity for industry leaders to get ahead by adopting and scaling computer vision solutions faster than their competitors. In doing so, they can improve field operations and increase efficiency, effectively lowering costs and speeding up deployment to outpace the competition. Companies looking to implement AI as quickly as possible are faced with the perennial question: do you build your own proprietary system, or should you buy it?
In this article, we’ll discuss what it takes to develop computer vision AI solutions in-house and provide insight into why buying a solution from a proven vendor is a better strategy for today’s growing network and utilities providers.
Thinking of Building a Proprietary Computer Vision Solution? Here’s What You Need to Consider
Typically, teams explore the option of building their own in-house software solutions when they want end-to-end control over functionality and performance. Leaders often believe that they need to build proprietary software to ensure that it’s tailored specifically to the organization’s operations. They may also think that in-house software offers greater data security and privacy—on-premises or private cloud solutions eliminate the need to send data outside the organization. The prospect of saving on licensing costs over time also makes building in-house an attractive choice.
However, achieving these outcomes with an in-house solution is more complex and costly than many leaders realize. The primary goal of using computer vision to increase efficiency and network or grid quality is reducing costs. But building—and later maintaining—a proprietary AI-based computer vision software is resource-intensive, lowering the cost-savings potential of implementing the solution.
Creating a Reliable, Custom AI Solution Requires a High Volume of Quality Training Data and Data Annotation
Machine learning (ML) model development begins with a high volume of training data. Teams have to gather and organize photos that reflect the images they need the model to analyze. Then, they have to annotate that data and indicate what the model should look for in images, such as operators’ names printed on fiber optic cables or objects surrounding a trench where infrastructure is installed. Collecting enough high-quality training data can be a challenge, especially if you don’t already have a workflow for capturing photos out in the field. One of the main reasons AI projects struggle to get off the ground is because organizations lack quality training data. In addition to the process of gathering and annotating data, tasks like model assessment and testing are time- and resource-intensive.
Benefit of Buying: Developing AI in-house takes time—from research and development (R&D) to testing and iteration—which means that the resulting models might no longer be a fit for the constantly-evolving business processes. With a robust platform like Deepomatic, you can launch model training in just a few clicks and deploy models rapidly so you can start driving results. Unit-T developed and deployed a new AI model in just a week using Deepomatic and quickly realized a 25% reduction in the time technicians spent on reporting out in the field.
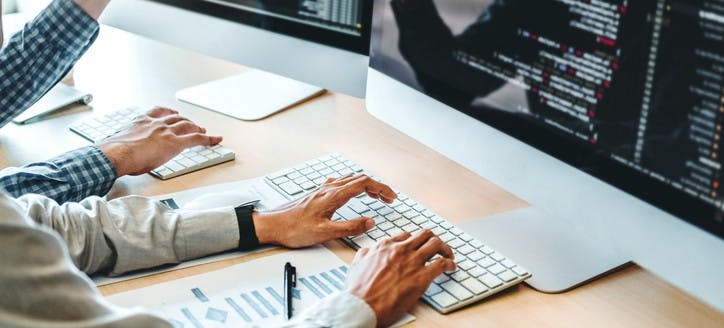
Building Machine Learning Models Alone Isn’t Enough
Creating an ML model that can accurately identify relevant information from the images your team provides is just the beginning. McKinsey reports that 90% of ML projects fail to deliver value because of issues with productization and integration into business applications. Teams must consider how their computer vision model will fit into real-world workflows in the field and in the back office. This might mean they need to leverage an API to connect to models deployed on cloud services, develop a custom integration with their field operation or network management software (e.g., FSM, GIS, or project management solutions), and create a proprietary portal or app to which technicians can upload photos. Teams also have to think about how to store the information that the model pulls out of the photos so that it’s accessible and useful, and how to keep data secure throughout the entire pipeline.
Benefit of Buying: Beyond developing a proprietary model, network and utilities providers often have to develop an entire platform. That’s where a solution like Deepomatic comes in. In addition to a custom ML model, Deepomatic offers frontend components and integrations with field solutions that make it easy to roll out intuitive computer vision functionality to your field technicians.
Developing a Robust Solution Takes Niche Expertise and Expensive Talent
When considering the cost of building in-house, it’s critical to factor in the costs associated with hiring new team members and paying their salaries—which can add up to over €1 million annually. Developing a computer vision solution requires the expertise and coordinated efforts of data scientists, DevOps, MLOps, and software developers. Organizations that don’t yet have individuals in these roles need to understand how bringing on and retaining talent over the long-term contributes to the total cost of the solution over time.
Benefit of Buying: Investing in a computer vision platform gives you access to a team of experts who already have experience building and deploying the type of solution you’re looking for. The annual cost of licensing is often much lower than the costs associated with hiring a team of ML specialists. The cost of labor is already looped into the price of a vendor’s solution. This can make it easier to forecast total cost of ownership and enable you to scale your solution without paying to scale your own team.
Keeping Up with Ever-Changing Field Operations Necessitates Ongoing Updates
While building in-house might eliminate licensing fees, you’ll still need to cover the significant costs associated with maintenance and updates over time. It may take years to develop an AI solution that meets your business needs. If your team faces competing priorities and shifts in business goals or processes during that time, they may not be able to keep up with the rate of change that must be reflected in the models they’re building. Field operations are always evolving as new equipment is introduced, operational workflows are optimized, and quality standards shift. This can cause data drift in the ML models. The solution will become obsolete if models are not regularly retrained to cope with those new field conditions. Leaders need to recognize that the solution they develop will need perpetual maintenance and take that into account when they estimate the total lifetime cost of building and updating their own solution.
Benefit of Buying: At Deepomatic, each of our data scientists deploys more than 2 models per week—velocity that few other companies can match. With Deepomatic, your team doesn’t have to worry about making updates to your models or creating new ones at scale. Our team provides ongoing maintenance so that you can stay agile and optimize your operations without compromising the functionality of your solution.
Buying a Computer Vision Platform is a Better Strategy for Network and Utilities Providers
Building an in-house computer vision solution not only comes with hidden costs, it also comes with significant risk. Developing a robust solution requires heavy, long-term investment in research and development, rigorous testing and optimization, and a clear roadmap to ensure that the models and workflows grow with your business. The complexity of highly functional AI-driven computer vision solutions makes them very challenging to build, contributing to the likelihood that what you develop might not end up working for your business.
Enterprise AI projects have a notoriously high failure rate. McKinsey has reported that only 36% of ML algorithms are deployed past the pilot stage. Meanwhile, Gartner predicts that 30% of AI projects will be abandoned in 2025 due to low data quality, inadequate risk controls, and rising costs. Recent industry estimates suggest that more than 80% of enterprise AI initiatives fail.
Fiber network and utilities providers are already busy with pressing priorities, including expanding their footprints to meet ambitious regional goals, increasing energy security and improving connectivity, and moving the needle on the green energy transition. Implementing a computer vision solution to improve field operations should support those initiatives through cost savings and increased efficiency. It shouldn’t reduce your organization’s capacity to pursue core objectives.
Choosing to buy a solution rather than build in-house means that you get a tested solution that can already perform well out in the field. You can realize rapid time-to-value and lower—or eliminate—the risk of investing in a solution that can’t deliver results. When you buy a solution, you can keep more of your organization’s resources dedicated to your revenue-drivers: deploying networks, building infrastructure, and winning customers.
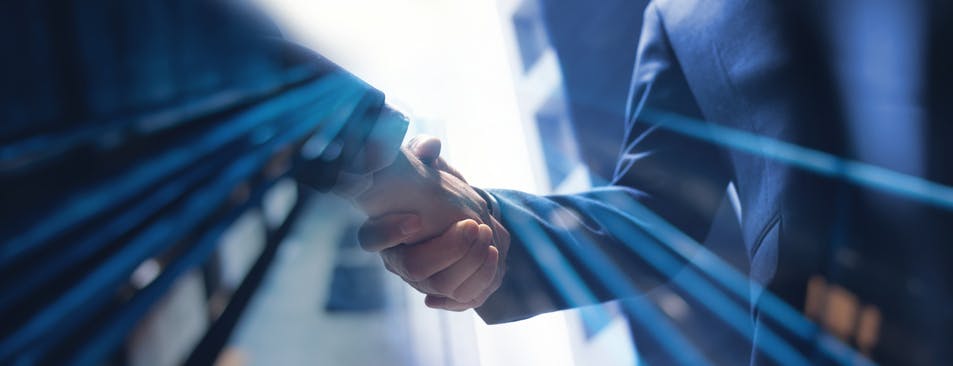
See 423% ROI on Your Computer Vision Investment with Deepomatic
Deepomatic offers a turnkey computer vision platform that can be customized to your operations and integrated with your existing field software ecosystem. The platform is purpose-built for telecom and utilities companies. Our team already has a robust data pipeline and off-the-shelf models that can be completed with models trained on data from your organization to ensure that the solution reflects your real-world field operations. We handle ongoing monitoring, maintenance, and updates so that the platform evolves at the same pace as your organization at scale.
With Deepomatic embedded in your field workflows, your technicians can simply take photos of the infrastructure or assets they’re working on and get real-time feedback to ensure they do the job right the first time. Using computer vision, you can eliminate the need for costly site revisits, reduce your spend on hiring quality control team members, and deploy more resilient networks and infrastructure that drive revenue over the long-term.
Network and utilities industry leaders like Vodafone Spain, Altice, Virgin Media, and more than 20 major operators across Europe and the UK leverage Deepomatic to streamline reporting and improve quality control in the field with computer vision. Organizations that choose Deepomatic have saved up to $755,400 in annual audit costs and seen a 423% return on their investment.
Deep dive into the business savings
Organizations that choose Deepomatic have saved up to $755,400 in annual audit costs and seen a 423% return on their investment.
Explore Forrester’s Total Economic Impact™ of Deepomatic
Book a demo to learn how Deepomatic can help you streamline field operations and give you unparalleled insight into the quality and efficiency of your network and infrastructure investments.