Lean AI :
How to create successfull computer vision systems in production
Deep Learning and its application to computer vision in particular has generated a remarkable amount of attention these past years, due to the vast range of tasks it applies to and the level of performance reached.
In this white paper, you will discover the result of our work and a new framework inspired by the recent trends in Agile, Lean and Devops, called Lean AI.
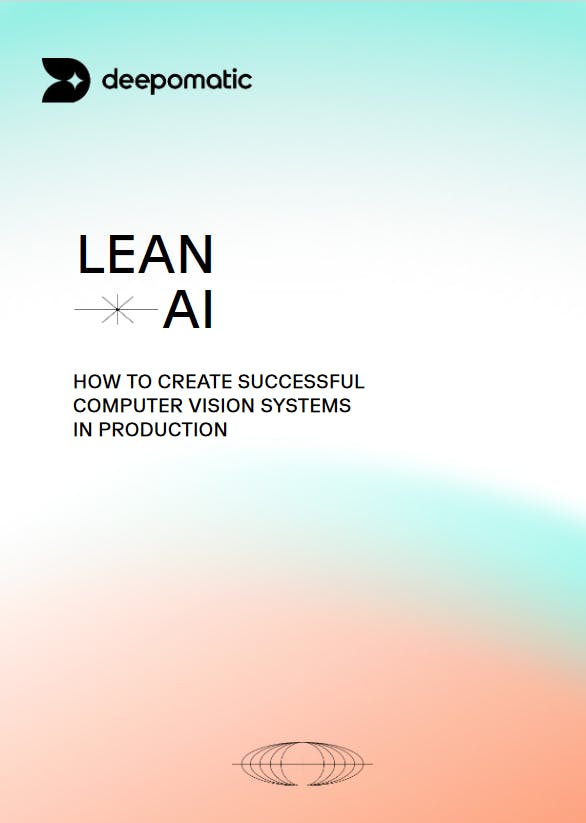