In 2021, Deepomatic was contacted by three marine biologists (1) working for Costa Humboldt, a non-profit organization dedicated to the conservation of Chile’s coastal and marine biological and cultural diversity. They were looking for a way to determine the abundance of the Juan Fernandez fur seal population and propose new management tools for its proper conservation in the future. Without thinking twice, Deepomatic immediately granted them access to their platform for free in order to demonstrate that computer vision could help monitor and manage biodiversity.
Partner’s Context & Challenge: Automate Data Processing
Geographic and historical context
The program took place in the Juan Fernández Archipelago. Situated 670 km off the Chilean coast, they are composed of three main volcanic islands. Island ecosystems, such as this one, are of great scientific value because they have served as natural refuges for multiple species throughout evolution and have a high amount of terrestrial and marine endemism.
Among all these species, one caught the attention of the organization: the Juan Fernandez Fur seal, whose proliferation is a cause of concern. The Juan Fernández fur seal was hunted indiscriminately between the 18th and 19th centuries, almost bringing the species to the brink of extinction. Rediscovered in 1965, its population has been sporadically recorded since. The first census counted 200 individuals, nearly 35 years later, there were about 40,000, and no accurate counts have been done ever since, but rough estimations suggest between 100,000 and 250000 individuals in 2019.
Tensions
This large and growing population puts pressure on the human population of the archipelago: The limited space available, particularly in the main island (Robinson Crusoe), is raising concern among the locals (approximately 1000 inhabitants). Furthermore, fisheries are the most important activity of the island, where six species of fish (around 120 tons/year) and two types of crustaceans are captured, including the famous Juan Fernandez lobster, which represents annual revenues of one million dollars for the local fishers. Today, it is uncertain if the large population of seals is starting to consume the same resources that fishers are exploiting, which could lead to conflict and increase the number of interactions between seals and humans.
Mission
To ensure a long-term balance, Costa Humboldt’s mission was first to perform a census of the number of fur seals as well as recognize the sex and the status of the individuals (female, male, juvenile, or pup), parameters that were not taken into account in several of the previous censuses. Their other goal was to evaluate the seal-fisheries interactions perceived by the community. Ultimately, their work aimed to propose management tools and strategies for the proper conservation of fur seals.
Challenges
Biologists have learned from nature for centuries through visual observation, gradually using drawings, paintings, and then photographs and video. Until very recently, population growth censuses were constrained by manual counts (also called “ground counts”).
However new opportunities arose from modern sensing devices such as drones and satellites, which are capable of taking aerial photos. Unfortunately, the manual interpretation of these photos is time-consuming and still subjective. Moreover, the data rapidly accumulates and researchers often face a data processing bottleneck and the potential of the data is not fully exploited.
This is exactly the situation in which the two biologists found themselves before getting in touch with Deepomatic.
Ecological data collected in the field presents a number of challenges that computer vision can help solve. The automated extraction and processing of information provides the opportunity to alleviate this long-standing problem. Here’s how.
Deepomatic Solution & Benefits: Challenges for Computer Vision Algorithms
As often in a computer vision project, the first step is to understand the data that Deepomatic is looking at and determine the best strategy to achieve their goal: differentiating and counting the fur seals on the images.
After granting access to Deepomatic Studio to Renato and Victor, they were able to upload their pictures, annotate them and together they trained various computer vision models. Once they were able to reach satisfying models to detect and differentiate the animals, they only had to run their model on all the images and add up the detections to reach the final counts.
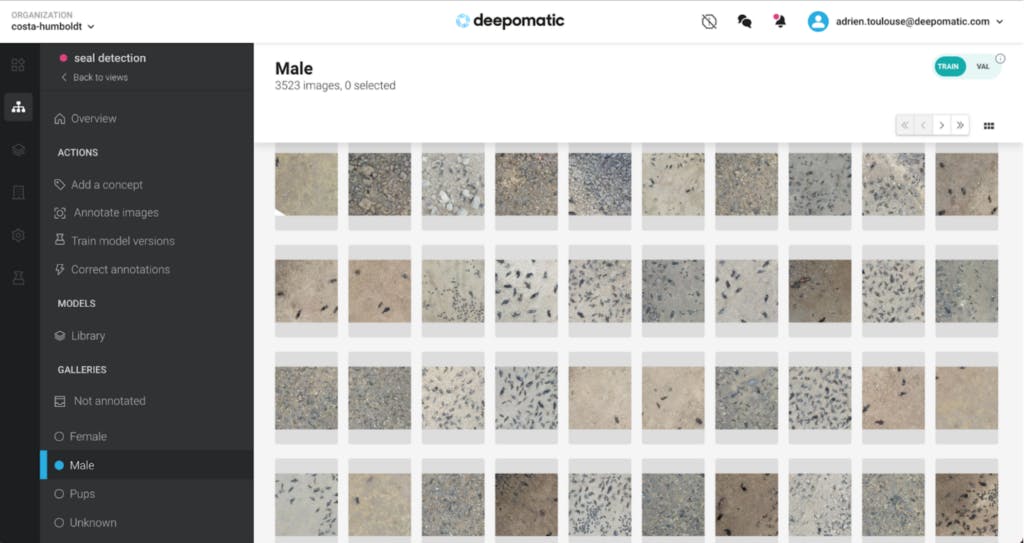
Fur seal dataset on Deepomatic studio
While trying to build a well-performing model, they faced numerous challenges:
- The diversity of pictures (the pictures stemmed from different locations with different backgrounds and were taken from varying altitudes with the drone. In case of bad weather, the pictures could also get very blurry)
- Elements from the background, such as wet rocks, could look very similar to fur seals.
- Differentiating fur-seal populations (the difference between juvenile and female is not always clear, especially in pictures taken from a drone)
These challenges demanded trying different techniques in an iterative process, such as the splitting of the datasets to gather similar images and build adapted models, the creation of new concepts to recognize elements looking like fur seals but which shouldn’t be counted, or the selection of the prediction with the highest score when two predictions were considering a single seal as both female and juvenile.
« From the very first models created from the training data, we already knew this would be a success. »Dr. Renato Borras, Marine Colector and Aquarium Coordinator
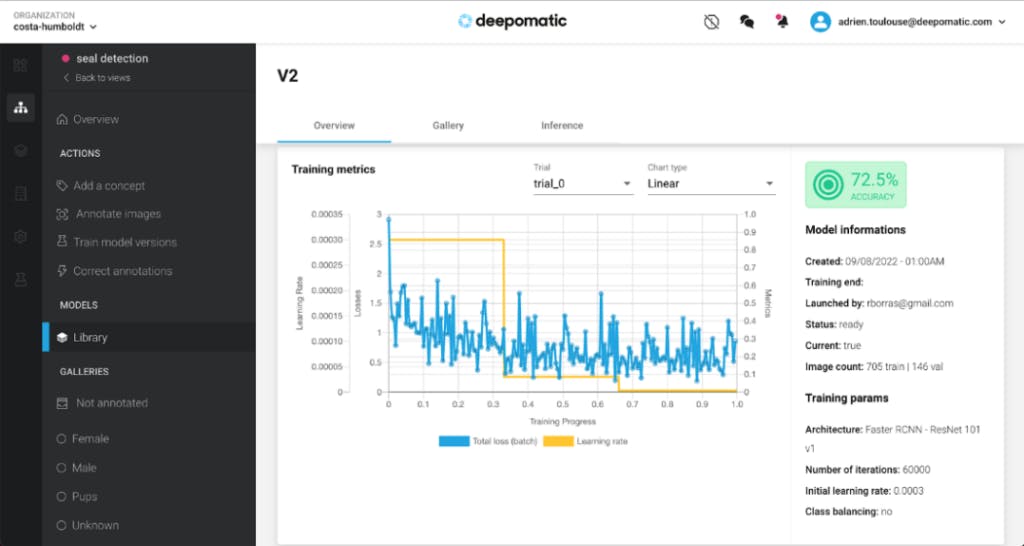
Building a fur-seal detection model with Deepomatic Studio
In this iterative process, easily visualizing the AI predictions directly on the platform was of great importance to analyze the weaker spots of their models and annotate new pictures to improve them or rethink their strategy.
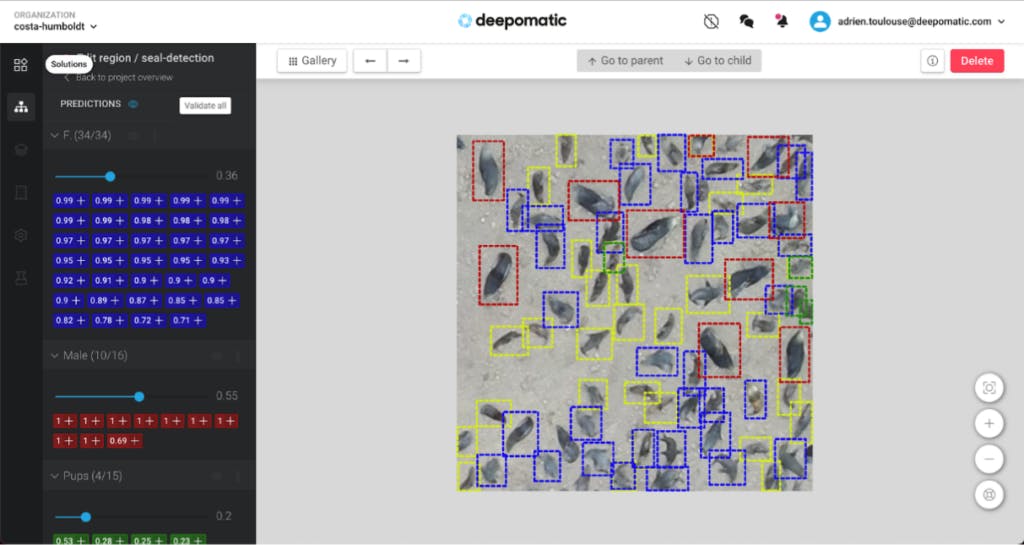
Visualizing fur-seals detections on Deepomatic Studio
Finally, their computer vision models allowed us to perform the count of about half of the total population of fur seals. The other half had to be manually counted on the platform due to insufficient data to train accurate models in some specific settings. Computer vision algorithms proved themselves to be the most efficient and powerful tool, given they were trained with sufficient quality data.
« The problems we faced had nothing to do with the tool itself but the correct collection of images. Once we refine the picture quality collection techniques, the problem will be solved. »Dr. Renato Borras, Marine Colector and Aquarium Coordinator
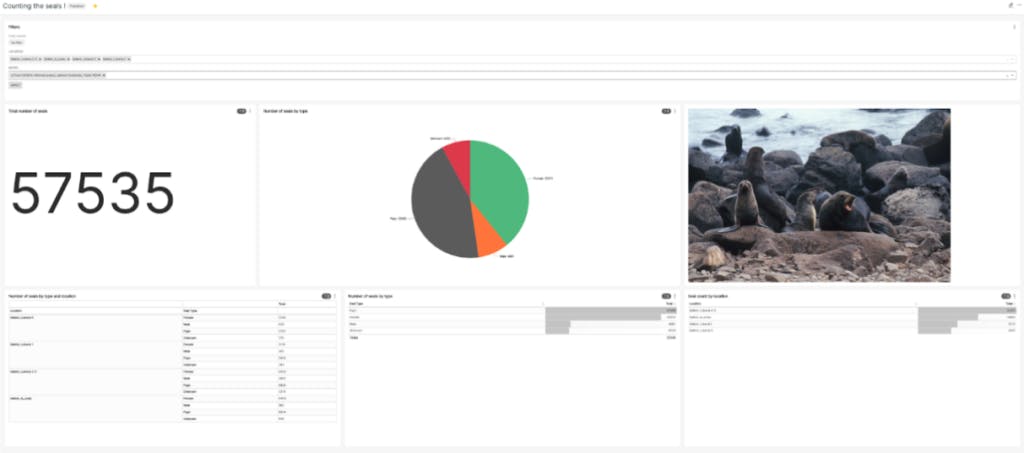
Example of automatic counts of fur-seals on Superset
In conclusion, technological advances in data collection, data processing, and data management are driving the field of biodiversity monitoring forward every day. The ability of computer vision to process large and complex datasets has led many researchers to explore how this technology can be used to help monitor their issue. Offering their platform to this program was a great opportunity to use their expertise for a scientific and impactful project.
If you have any questions about the project, please contact: lobofinojf@costahumboldt.org
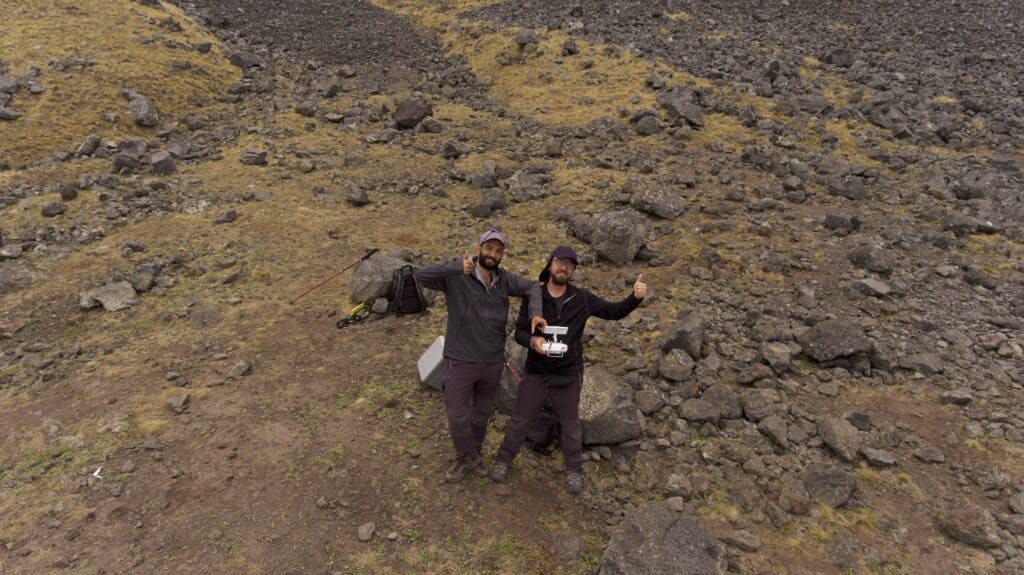
(1) Dr. Renatos Borràs-Chàvez, Victor Castillo & Luciano Hiriart-Bertrand